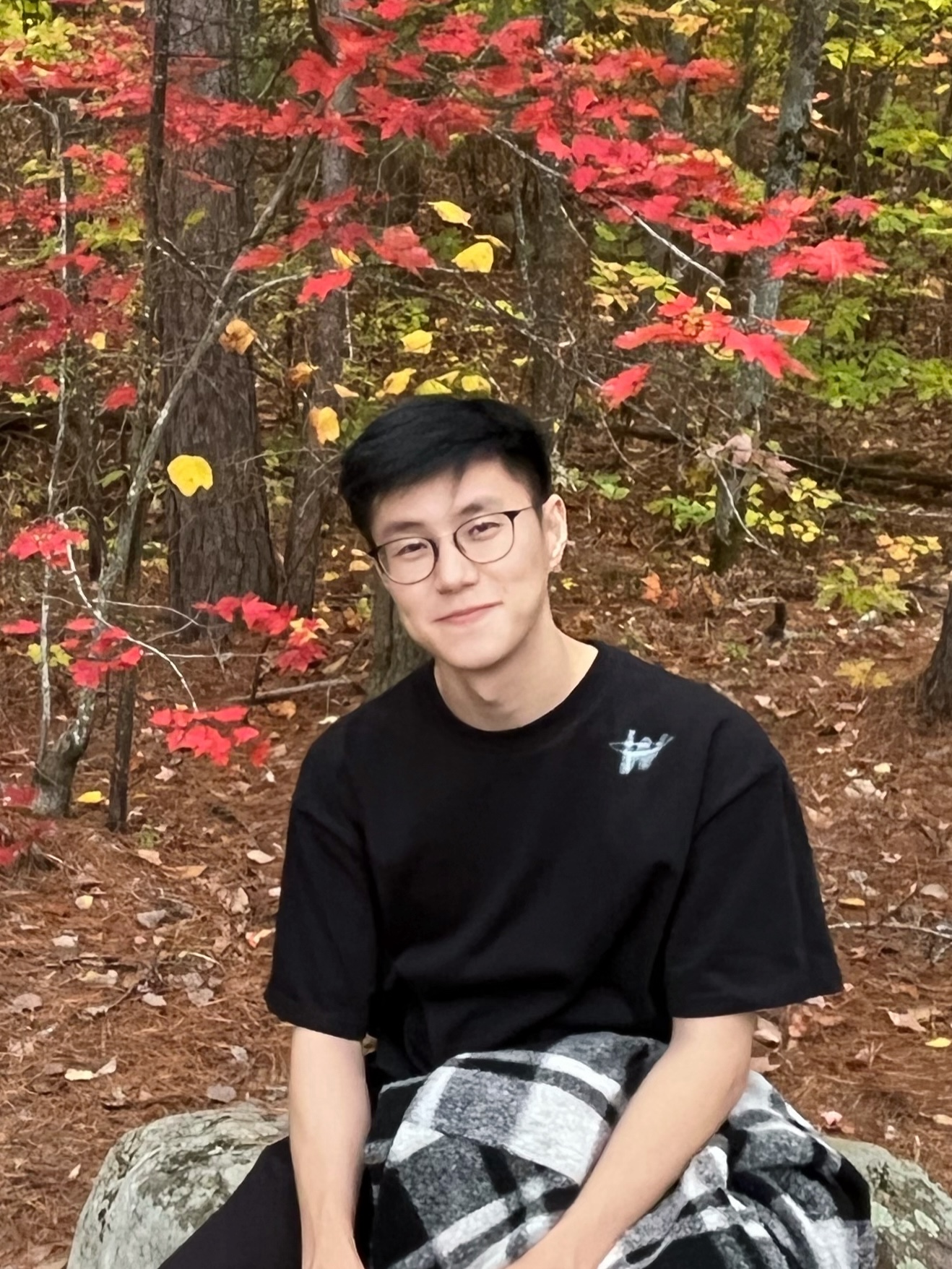
Dissertation Title: Social Interaction and Activity Space in Urban and Rural America
Committee: Kathleen Cagney (University of Michigan, co-chair), Luc Anselin (co-chair), Christopher Browning (OSU)
How do individuals navigate through different spaces, and how are their movement patterns and "choices" shaped by the social contexts in which they are embedded? My dissertation examines human mobility and patterns of activity space - the collection of locations to which individuals are exposed on a regular basis. Using a range of unique mobility data, including GPS location tracking from probability samples, transportation surveys, and big mobility data of mobile phones and connected vehicles (or "found" big location data), I explore how micro level characteristics (e.g., race/ethnicity, socio-demographics, perceptions) and meso level social context (e.g., residential segregation and clusters of segregation, rurality/urbanicity) structure mobility patterns and routine spatial choices. Drawing on the literature on neighborhood choice and decision science, I am interested in understanding whether, through carefully designed nudges, behavioral adaptations among residents of both segregated and non-segregated neighborhoods can be an effective strategy for social integration. My work integrates perspectives from urban sociology, geography, decision science, criminology, and more.
My research asks how human behavior (esp. mobility and crime related) is shaped by social and physical contexts and how causally relevant contexts should be defined. My recent work has explored activity space exposures, income segregation around the clock, and spatio-temporal patterns of crime. My ongoing projects examine 1) the spatial decision-making process and its social context in activity space, 2) how mobility patterns factor into individuals’ rural/urban living experiences, and 3) how social scientists can leverage computer vision AI to decipher local social ecology from street view images.