Finding political leanings in large language models
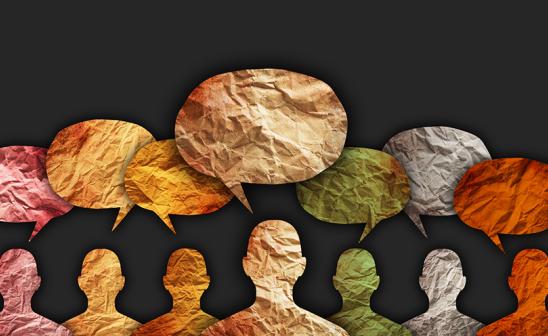
UChicago and UC Berkeley researchers develops methods to dial societal consequences down (or up) for research and practical use
By Sarah Steimer
New studies led by UC Berkeley and UChicago researchers explore political leanings in large language models (LLMs) such as ChatGPT. Within their findings, the researchers say that political neutrality in LLMs might not be the right approach; rather, a greater range of LLM perspectives can enhance the diversification of voices.
Their first exploration of political leaning in LLMs was a study published at Empirical Methods in Natural Language Processing (EMNLP), a top natural language processing conference in 2024. James Evans, the Max Palevsky Professor in the Department of Sociology, says the motivation of the study was to better understand to what degree LLMs are natively and culturally leaned toward one perspective or another, where that leaning originates, and what the consequences are.
The research team — which included Evans, Dawn Song (UC Berkeley), Yujin Potter (UC Berkeley), Shiyang Lai (UChicago), and Junsol Kim (UChicago) — used a voting simulation to show 18 open-weight and closed-weight LLMs’ political preferences for Joe Biden (and later Kamala Harris) over Donald Trump. They also found that the Biden-leaning became more pronounced in instruction-tuned models, compared to their base versions. This suggests that the process of post-training may have heightened the left-leaning tendency.
“It's likely because they're training on things that are implicitly correlated with left-leaning (ideas): inclusiveness, positivity, nontoxicity, and even accuracy,” Evans says. “If you train them to behave in this nonjudgmental way, all these different factors that are cultural ideals for the left, then they also become politically left-leaning.”
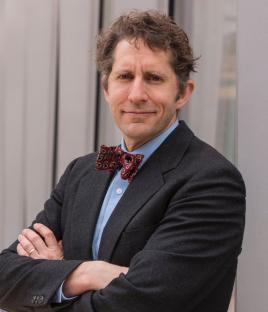
The team then explored the potential impact of LLMs on voter choice: 935 U.S. registered voters interacted with LLMs (Claude-3, Llama-3, and GPT-4) over five exchanges each. These political conversations covered both candidates’ policies, and although LLMs were not asked to persuade users to support Biden, about 20% of Trump supporters reduced their support for Trump after LLM interaction. Many users also said they had a positive exchange with the bots and expressed a desire for further interaction with LLMs on political subjects. It remains unclear why the LLM interaction affected voters’ political stances.
As a social scientist, Evans says, a natural next step is to explore the empirical impact of LLMs with these and other leaning tendencies.
“There are hundreds of millions of online bots people use,” Evans says. “Increasingly, they're used in all sorts of settings, and play more and more roles in society, from structured tasks like triaging your call to an airline to unstructured conversations, as with therapists and tutors. People are going to be talking with them about all kinds of things, from intimate content to voting choices. Our thought was: Let's project ourselves into the future. Insofar as people are going to be interacting more and more with these bots, and if they have an opinion, to what degree will that opinion matter?”
The blog to be presented at the International Conference on Learning Representations (ICLR) in 2025, written by Potter, Song, Yejin Choi (UWashington), and David Rand (MIT), also discusses the path forward and open questions to better understand the impacts of LLMs on our democracy. They propose research questions in four broad areas: (1) evaluation of LLM political leanings, (2) understanding LLMs’ influences on our democracy, (3) better policy frameworks for AI development, and (4) technical solutions to mitigate political leanings. In particular, they consider how controlling political leanings of LLM outputs remains challenging.
Some studies including recent work by AI company Anthropic, the EMNLP paper, and the forthcoming study to be presented at ICLR in 2025 have begun addressing this technical challenge. In Anthropic’s work, they applied sparse autoencoders to extract a neutrality feature to make LLMs more politically neutral. In the EMNLP paper, the authors showed that by applying representation engineering, one can make LLM outputs more politically neutral, utilizing a linear representation of political ideology in models. Similarly, Evans, Kim, and Aaron Schein (UChicago) found that LLMs possess a linear representation of American political ideology. In the ICLR paper, they identified, monitored, and steered the political slant of their output along the broad spectrum of American political ideology (i.e., not only toward neutrality but also more liberal or more conservative ideology) by leveraging this linear representation.
“We found that there is a kind of signal in the AI we can detect to know whether it’s being slanted toward the left or right,” Kim says. “We were able to audit and measure the political perspective implicitly adopted by the AI. We also found that we can use those signals to steer the slant of the AI's textual output.”
The researchers intervened in a set of attention heads in the LLMs that represent the U.S. ideological slant. Through this approach, they were able to tune LLM output to any position along the linear dimension from a liberal to a conservative ideological perspective. It worked like a dial: They could turn the vector to a neutral space, or turn it to be somewhat right- or left-leaning.
Having this control has two major potential uses. First, it can help simulate political debates among individuals with diverse political leanings. Before political platforms or statements are presented to the public, they could be tested with AI that is programmed to various parts of the vector space to see how the discourse could play out and where potential conflicts could arise. Second, it could help with diversification: Studies have shown the benefit of diverse opinions for a host of creative tasks, but it’s not always clear what the best mix might be. LLMs with different personas or political leanings can be brought together to find the right cocktail. For example, someone could brainstorm or test political or creative ideas with an LLM.
“Can we do better as people in any creative or productive domain by creating the right ensemble of perspectives?” Evans asks.
The researchers continue to fine-tune their understanding of political leanings in LLMs, and how those political leanings can be beneficial — which largely revolves around greater diversification.
“Our assumption is not that these bots shouldn’t be biased,” Evans says. “But maybe there should be an unbiased distribution of bots that people engage with so that they can have choices and draw upon them as resources, rather than feel like they're being manipulated by them if they all share the same view.”